Unveiling the Secrets: How Large Language Models Revolutionize MLOps for Data Wizards
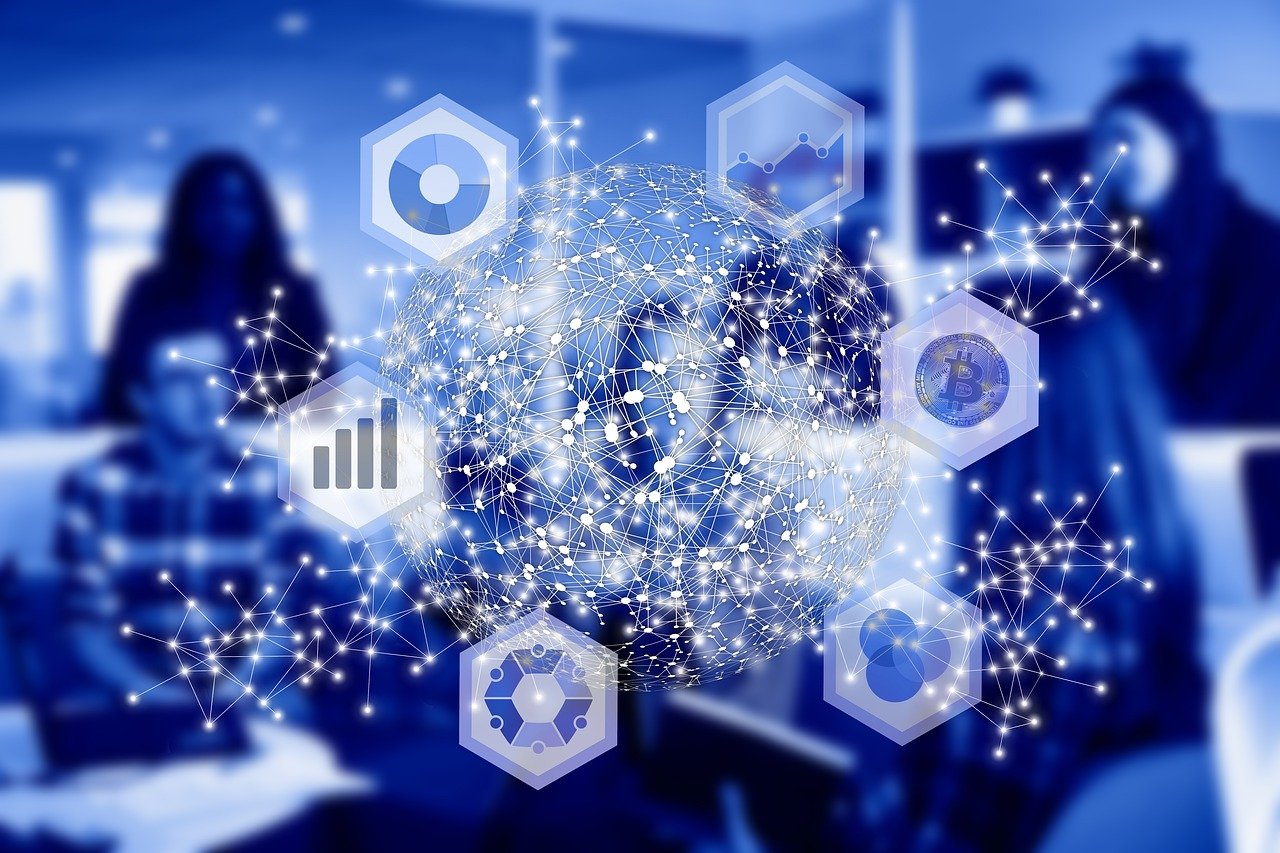
Data science is the crossroad where methodology and magic coincide, enabled by a squad of modern-day sorcerers or the data wizards. They conjure insights from an ocean of data, and their equipment just received a powerful upgrade.
In this golden age of data-driven decision-making, where every bit of information is valuable, these data wizards use language models like GPT-3 as their wands. This domain, which was once reserved for the highly tech-savvy, has now been democratized.
How, you ask? Now, this domain allows even the unversed to fluently converse with machines, instructing them in the mysterious language of data.
In this wizardly voyage, let’s navigate data accessibility, how it is accelerating the pace of decisions, and automating the once-grueling tasks that data wizards grappled with. Let’s also extract the powerful spells wrapped around complex data, transforming data wizards into alchemists who are capable of modifying raw data into highly valuable insights.
Apart from the above topics, we will also tread through the path of ethics and responsibility. Because with the great power of Large Language Models (LLMs) also comes great responsibility.
Are you prepared to witness the transformation of data wizards into modern-day fascinators? Let’s unveil!
What Are LLMs?
LLMs, short for Large Language Models, are a category of artificial models based on neural networks that are designed for natural language processing (NLP) tasks. Their defining traits include immense size combined with complexity, and they often contain innumerable (millions or even billions) parameters (learnable elements).
What Are Machine Learning Operations?
Machine Learning Operations, commonly known as MLOps, are practices and tools to streamline and automate the end-to-end machine learning (ML) lifecycle. It starts from model development to deployment and supervision. It is inspired by DevOps- used for software development. However, it adapts its principles to the challenges that machine learning presents.
The Impact on Data Science
The advent of LLMs has fundamentally changed the entire scene of data science and MLOps. By democratizing access to machine learning and allowing individuals with little to no coding knowledge to experience the power of advanced models, data wizards have eased communicating with these models using everyday language.
Moreover, LLMs have sped up the advancement of NLP applications. Convoluted tasks such as chatbots, sentiment analysis, content generation, and text summarization have seen extraordinary progress, opening up new avenues for data mavens.
Fascinatingly, LLMs have extended their impact beyond NLP. They have demonstrated their expertise in various domains, from rather easy tasks like image generation to solving complex scientific problems. This flexibility is rewriting the boundaries of what can be achieved with machine learning.
Now, let’s delve deeper into the secrets of LLMs and their transformative effects on MLOps in the context of data wizards and their data-driven insights.
How Are LLMs Transforming MLOps for Data Wizards?
LLMs are enabling a drastic change in MLOps for data wizards through several technical developments and competencies. Let’s explore them.
Natural Language Interface: LLMs impart a natural language interface to enable interaction with machine learning systems. Data wizards can use simple language questions and commands to instruct and interrogate models. This eliminates the need for extensive program design or knowing domain-specific languages.
Automated Data Processing: Traditionally labor-intensive and time-consuming data processing tasks can now be automated with LLMs. Not only do they excel in data cleansing and feature engineering, but they can also do data summarization.
For instance, LLMs can automatically identify and handle missing data, outliers, anomalies, or even inconsistent values in a dataset, thus reducing the need to put in any manual effort.
Rapid Prototyping: Data wizards can utilize LLMs for rapid prototyping and research. They can quickly generate codes or data transformations to test theories and discover data, allowing for relatively faster iterations in the process of model development.
Natural Language Reporting: LLMs can even lead the automatic generation of natural language reports and elucidations for model outputs. This helps the data wizards explain complex model results to even the non-technical stakeholders, helping foster better collaboration and understanding.
Data Integration: LLMs can aid in integrating data from numerous sources by understanding and interpreting data schemas and formats. They facilitate the merger of heterogeneous datasets, which is a common challenge in MLOps.
Streamlining Data Labeling: Data labeling is among the most critical tasks in supervised training and learning. It is also a strenuous task. LLMs can help in automating certain parts of the data labeling process. Therefore, they can aid in reducing the manual sweat required to annotate training data for machine learning models.
Real-time Insights: Leave it to LLMs to process and analyze textual data in real-time. This significant skill enables data experts to gain immediate understanding from streaming data sources, thus making it possible to react instantly and swiftly to evolving conditions or events.
Efficient Collaboration: LLMs can also enhance the collaboration between data experts and other team members. They can help in the automatic generation of documents, code comments, and explanations, making it smoother for the other team members to comprehend and work with the models developed by the data experts.
Continuous Learning: They support continued learning by providing updated information from an extensive range of sources. This allows the data experts to stay informed about the current ongoing research, trends, and data sources related to their work.
Automation of Model Deployment: LLMs can assist in the automatic deployment of machine learning models to production environments. They can generate scripts for deployment, containerize models, and handle version control, hence simplifying the deployment process for data wizards.
Enhanced Monitoring and Debugging: Supervising and debugging machine learning models in production is crucial and LLMs can assist in that. They can mechanically detect anomalies or drift in data distributions and then trigger alerts to data experts so they can take appropriate action.
Reduced Barrier to Entry: Reducing the barrier to entry for those who may not have extensive expertise in machine learning or programming is an essential task that LLMs do. Data wizards can leverage these models to execute advanced data analysis and machine learning tasks without having any skilled or deep technical knowledge.
Ethical Considerations
In this landscape, ethical considerations stand as an indispensable compass. As LLMs play a gradually pivotal role in data-driven decision-making, ensuring responsible practices in Artificial Intelligence (AI) becomes paramount.
Ethical concerns include a wide spectrum, from bias mitigation during model training to clear disclosure of AI-generated content. The test lies in addressing issues of fairness, accountability, and privacy, especially in domains where LLMs wield substantial influence.
Methods for adversarial testing and robust assessment are crucial to identify and rectify bias in LLMs, while data anonymization and consent-driven data collection become imperative for the protection of individual privacy.
What Does the Future Hold?
- The future unfolds with the promise of even more profound advancements. LLMs are composed to become integral components of the data science ecosystem as they can seamlessly integrate into existing infrastructure and workflows.
- Future iterations of LLMs will likely exhibit heightened contextual understanding and will enable much more nuanced interactions and more accurate responses.
- In addition to this, LLMs will continue to grow in terms of their capacity to handle multimodal data, combining text, images, and audio for a holistic understanding of information.
- The future will also see increased emphasis on fine-tuning LLMs to specific fields and domains, which in turn will enable data wizards to harness their power for industry-specific tasks.
- However, as we step into this future, ethical considerations and responsible AI practices will remain at the forefront, guiding the development and deployment of LLMs to ensure transparency, fairness, and respect for privacy.
Concluding Thoughts
In the vicinity of MLOps, the unveiling of LLMs as transformative tools for data wizards heralds a future where data operations and machine learning reach new heights of easy accessibility, user-friendliness, and efficiency.
This journey reveals that LLMs have transcended the status of mere tools and become catalysts of innovation and collaboration. With their natural language interfaces, LLMs have democratized machine learning, allowing data wizards and experts to interact seamlessly with complex models.
Moreover, they have accelerated decision-making, automated data tasks, and empowered data experts to tackle multifaceted challenges.
However, as we navigate this pathway where technological advancement wins, the challenge remains in ethical considerations, guiding us toward responsible AI practices and ensuring that the fresh power of LLMs is harnessed judiciously for the betterment of society.
The future calls us with the promise of even more profound transformations, where LLMs become all-pervasive enablers of data-driven decision-making.